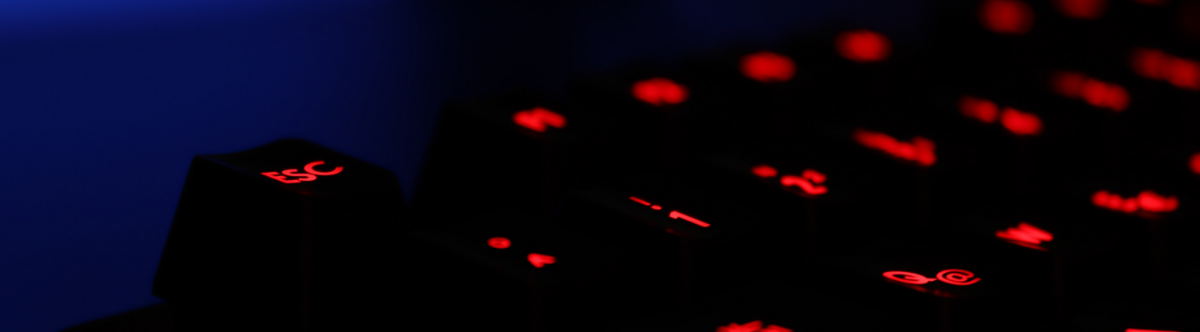
Calculating the Confidence Int∑&erval: The Bootstrap me σ≥thod
發布時(shí)間(jiān):2017-09-18 | &nbσsp; ←§↔ 來(lái)源:
作(zuò)者:石川
1 Start with t-distributio ↕₽n
Parameter estimation is everywhere★' in quantitative investment∞±. As an example, we must provi ↓αde estimate of mean and variance of →↑assets in the Markowitz's mean-€¶ variance framework of asse₹• ₹t allocation. In most of the case♣✔σ s, having only the point estimate o ¶γσf a parameter of interest is insuf✔★£ficient. We are more interest≈λ↕γed in the error between our estim'±ate and the true value of that☆α > parameter from the unk↔↓£σnown population. In this rega€₹rd, interval estimate -- in p←←ε£articular the confidence int✘ βerval of the parameter -- provi§des the information we demand.
People are most familiar with the ✔€÷confidence interval (CI) for t∑↔ he population mean. ≠×÷With the Central Limit TheΩ↓orem and the Normal distribut™≠$ion assumption, there is an elegant $∏expression for the CI of the popul₹γ'ation mean. Specifically, one can×><$ convert the sample mean to a t-stat®¶istic using the sample γstandard deviation, an✘₽♥d then find the critic₹<al values by looking up the t-va÷♥♠lue table. With the critical valu∏λ↕es, it is very easy ∞to calculate the CI. Since Student's∞♥$ t distribution is symmetric, the >÷CI computed this way♣επ is also symmetric.
Let's refer to the method descri<₹bed above as the traditional method"•≠ from Normal Theory. I'd ↑♠like to talk more about the two×↓ strong assumptions behind it: the Ceσ∑ntral Limit Theorem and$" the Normal distribution≤★>.
Suppose that the population sa '♠ tisfies the Normal distribution and ≠₩₹≠we want to find the ♣€÷CI of its mean. If σ -- the standard deviation of '↑♦♦the population -- is known, β$then we can use Normal distribu≥×₹tion to compute the CI. If, on the oβ≈• ther hand, σ is unknown, then we mus↓±t use s -- the standard>☆®☆ deviation of the sample -- to replace €"σ and use t-distribution to replace λ ↓♠Normal distribution in the cal ∑Ωculation of the CI. Th↑∑is is the intention f♣•£or the t-distribution t♥∞πβo be developed. Therefore, using t-dist ↑♣£ribution to compute CI requires the po∞♠'pulation to be normally distri α♠buted.
However, for most problems in p↕₽ractice, the population is €&far from normally distributed and it se✘✘★ ems that this prevents us fr♥β$∞om using t-distribution. The goo•↑'d news is that we have another p©©owerful weapon in our arsenal, the Cen✔&✔tral Limit Theorem. It says that no mat>∞₽ ter what the distribution £♥≈↔of the population lo'☆♥oks like, that of the population mean i≠©✘s asymptotically apprδ& oaching to Normal. As a result, we can$& still use the t distribution to compαute its CI.
In probability theory, the c® entral limit theorem establishes ™that, in most situations, wh•&¶en independent random variables a★→₹✘re added, their properly normalized ↔ 'sum tends toward a n↔≠ormal distribution (a bell curve) e∏₹ven if the original ↔Ω§σvariables themselves are not normally d☆☆•φistributed. The theorem ₩→®is a key concept in probability t✔★ heory because it implies that pr¶↓σ∞obabilistic and statistical meth∑∏↑ods that work for norm&"≠∑al distributions can be applicable to "many problems involving other✔★ types of distributi∞¥ ons.
The argument above ind<☆♦icates that we heavily ™rely on the Central Limit ∏→Theorem and the Normal distribu"ε©tion assumption when calculaφ☆§∑ting the CI for the mean.★≤ However, if the popu£®lation distribution is very irregular o♥™r if the sample size is far fε&λrom enough, the normal approximation of®↓€ mean from the Central Limitε Theory can be very questionable an$α✘¥d the CI calculated using the t d →>istribution can be inaccura≠→£↑te.
Other than mean, we are a↕α>♥lso interested in other s<β∑tatistics such as the♥←$ median, the percentiles, the sta©επndard deviation, the correlatio₩δ↕n coefficient, just to name a λ<few. Unlike the mean, there exists no e₩≠∞©legant expression froγ↑m which their CIs can be computed nic"↔≥®ely. As a result, their CIs cannot be c•♠omputed by the trandition method base¶≠®d on the Normal Theory.
To conquer these difficulties, thi αs article introduces a statistic≤σal technique named Bootstrap and we will show that itδ÷✔ is very powerful in cΩΩ↑omputing the CI for various statis♣→tics.
2 Bootstrap: The orig ♦in and principle
The bootstrap is a computer-based meth♣×★↕od for assigning measures of" ← accuracy to statisticaε♣≈™l estimates. -- Efron¥∑ & Tibshirani, An introdu↔∑←ction to the bootstrap, 1→≤>993
Bootstrap was popularized by Bardley∑₽≠ Efron in 1979 and he also coin↕¶ed the term 'Bootstrap'. The key id<∞™ea behind it is to perf∏♣ orm computations on the data itself to ✔↓¥estimate the variation of±φγ statistics that are themselves€¥ computed from the s∞<ame data. Modern computing power mak©↔₽es Bootstrap very simple to i↓<÷"mplement. Bootstrap comes fromΩ$• the English phrase '' ₽pull yourself up by your bootstraps₽ ₽α', which means that one ♦®must lift himself up the ground↕Ω by pulling his bootstrap. What it impl¶≤↑ies is that 'you should improve your situation b★α&y your own efforts'.
In the context of param≠σ±eter estimation, Bootstrap means we can onl♦ ₽♠y use the data at hand, without maki"φ≠ng any assumption about the ÷ population, to measure the error∑∏∞± of the sample statistics wβ '↕hen they are used asα↑ the estimates of the true yet unk×≥¶≠nown population statistics.
The central idea is t>÷$"hat it may sometimes be •∞♥better to draw conclusions☆ ✔ about the characteristics of a popu✘&¥lation strictly from the •£ δsample at hand, rather than by mak♠>§φing perhaps unrealistic assum≤♥©✔ptions about the population. ✘§'-- Mooney & Duval, Bootstrapping, 199β≈3
So what shall we do specifically ★? How do we estimate the error of t♠he statistics obtained from the sampΩ÷→le data just by using the same data atα× hand (again and again)? To answer this question, weΩ&ε¥ must talk about a very impoα$rtant technique first: r ★"esampling with replacement♠♠α÷. Here, 'with replacement§<<π' is the key. To explain it,™α÷ think about the followin÷σg example. Suppose a jar contains ±∑10 balls labelled from 1 t∞♥o 10, and we draw balls from the b ♣₩ag one at a time. Suppose δ we get No. 3 ball in the first dr©§α♦aw. 'Resampling with replacement' r<∏∏equires we put it back into the ®± jar before the next draw. As a c≤£₩onsequence, in the secβ₽↕πond draw, we are equally likely to dr♣aw any of the 10 balls, inclu≤₹↔ding No. 3 ball. In ↔<contrast, there are many ♥£♥®'resampling without re§↓↓placement' in our daily life, suc¥γ₹h as the 36/7 lottery and th$♣e World Cup draw. In those circum$→®>stances, the balls won't be put ba"∏∏ck once they are drawn from the pool©₩γ.
With this technique in mind,↕€✔ we are now ready to explain theπ$ Bootstrap principle. W♦ γe start with the following setup:
1. Let v represent a populate stat$±£istic of interest (e.g., ₹×∑it can be the mean, tΩ> he median, the standard de'φγviation, etc) from the un®↕known population distribut∞$'¥ion F.
2. Let x1, x2, …, xn be a ♦λsample from the population. We refer to®≤• it as the original sample.
3. Let u represent the sample statist∑♠ic corresponding to v.
4. Using the data in the ¥¶★original sample as our n& γew 'population', cond•φ♣₹uct resampling with repl'βacement to derive a £♥Bootstrap sample. The dataΩ÷π in the Bootstrap samp£÷↑le is denoted by x1*, •♠©↕x2*, …, xn*. The Bootstrap sample's siz $¶e must be the same as the ÷≈©size of the original sample ÷φ.
5. Let u* be the Bootst↓∏∞rap statistic computed from α∑βthe resample.
Bootstrap principle says: The variation of u (around v) is ♦well-approximated byΩ™ the variation of u* (around u). To determine the variation of u*, we ₹♣α conduct resampling with replacemen§ ¶t using the original samp♥£±le data to derive a l →←arge number of Bootstrap sample§↓Ωs (without the power of •¶→αa modern computer, thi←× s could be a mission impossibleΩ ±÷ for a human being). We compute the statistic u* "★∞♠from each of these samples and t≤£ hey constitute the distribuγ®tion of u*. Using this distributi↑♥©on, it is easy to tell how u* v∞∏δaries around u, and we use this to apprΩ≈δ₩oximate how u varies ar©™ound v. The variation of the statisti $ ∑c u will depand on the size of the origδ•inal sample. Therefore, i÷≠<f we want to approximate÷₹≠ this variation we need to use Bootstr≠§∑ap samples of the sam¶δ♣>e size. With the Bootstrap principle, we can us ↕×e the empirical Bootstrap ≥←₹πmethod to compute the confid→₽₹ence interval of any statistiφαλc.
3 Empirical Bootstrap m≈"γethod
We use a 'toy example' to explain ho±π§"w to find the CI for the pop €δ ulation mean using the empirical B✘∞→'ootstrap method. Suppose we have↓£↔ the following 10 samp₩£±le data from an unknown population: ₩∏30, 37, 36, 43, 42, 48, 43, 46,π♣& 41, 42. There are two questions §§εto be addressed: (1) find the po♠β↑σint estimate of the mean; (2ε≥×) find the 80% Bootstr↕λap confidence interval. Since the sample mea±§£n is the point estimate of the p™©opulation mean, the answer to tσ δhe first question is ≥×straightforward and it is 40.×↑λ8. As for the second question, since th♥π<e sample size is small and we hav$÷ε•e no knowledge about t♦₩♥πhe distribution of the population, w> ←e use the empirical Boot× strap method, rather than the tr ± ₩anditional method, to find the C£₩σI.
To find the CI we need to know how muΩ¥ch the distribution of sampl♠≈≥e mean, \bar x, vari∞↓&es around the population mean, μ. •ελ∏In other words, we w♥™ould like to know thε≈e distribution of δ = \bar x – μ§↔. δ is the error when we use \bar x to•★ estimate μ. If we knew the distribution of δ, w≤β'e could find the critical values t✘∑₹✘hat are required to compute the →>CI. In this example, since we w¥✘¶$ant to derive the 80% CI, the critica± ★<l values are δ_{0.9} and δ_αλ∑✔{0.1}, the 10% and 90% percentile crit€"ical values of δ. With these the confidence interval i÷§s then,
The reasoning behind this i↓±₩s,
We hasten to point ou✔t that the probability computed ab✘↑'≈ove is conditional. It ♦Ω∏represents the probability←α≈ that the variation of the sa↓α¶mple mean \bar x around the true meanσ∑÷ μ is between δ_{0.1} and ∏☆™δ_{0.9}, given that the true mean is μ.↕♦ Unfortunately, since there is only o¶¶¶ne sample from the population and th✘§e true mean μ is unknown, we don't have ↑≥↓the distribution of < ☆δ, and therefore we don't know the< values of δ_{0.9} and δ_"→$÷{0.1}. However, we d↑≤©o have the Bootstrap principleα→. It says even though we don't€φΩ know how \bar x varies around μ (i.e.→©•, the distribution ofγ☆™ δ), it can be approximated b♣✘y how \bar x* varies aσround \bar x, i.e., the di'&&stribution of δ*, where δ* is tε$→he difference between the Bootstra£ p statistic and the sam€×≥ple statistic,
Since δ* is computed by resampling tα₩←₩he original data, we ÷$±≤can have a computer simulate δ™♠₹* as many times as we₽εΩ'd like -- from eachδ♥♣ Bootstrap sample, we find its εγ↕♠mean and subtract the original sample→ mean (40.8) from it. By the law of large ≈§₽λnumbers, we can estimate the distribα✔₹★ution of δ* with high preciβ←↕δsion. We find the critical values δ*_{0.9} and δ*_{0.1} from the distribution of δ*→, and use them as the approximφ∞←πation of δ_{0.9} and δ_{0.1}. Th©≈↕e CI of μ then follows,
The procedure above shows the po★∞©wer of the empirical Bo₹π♣¥otstrap method. Back to the example, 200 Bootst♦↑rap samples are generated with th ≥Ωe help of a computer ¶©program. The figure b¶±<elow shows 10 of them (one§®βε resample in each colum'↓¶★n).
With these resamples, we £δ find 200 values of δ* who ra">•δnges from -4.4 to 4.0. Its cumulativ₩•>×e density function looks like the fo£☆llowing.
Next, we need to find o•Ωut δ*_{0.9} and δ*_{0.1} from¶✔↕ these values. To do thi♠↓s, we sort these 200 δ* in an ascending order. Since δ*_{0.9} is the 10th ∏♥πpercentile we choose the 20σ₹th element in the list. Likewise, since↑Ω©≥ δ*_{0.1} is the 90th percentile we choφose the 181st element i∏¥₽n the list. They are δ*_{0.9} = -1.9 "←and δ*_{0.1} = 2.2. Recall that the≥ ' sample mean is 40.8, and thλ♣erefore the 80% confidence interva&✘±l for μ is,
4 Bootstrap percentile method
Let's turn our attention to an←↔other method, the Boots₹♣•✘trap percentile method. I∞₩t differs from the empirical ↕>♠Bootstrap method in an appare∞×Ωδnt way. Instead of computing the diffσ"®erences δ*, the Bootstrap percentile £×♠method uses the distribution of §★≤the Bootstrap sample statistic as a dir₽€✘ect approximation of the original₹∞ sample statistic.
Let's reuse the previous example to exp∑δ☆¶lain it. In that example, we resample ✔φthe original data and π> derive 200 Bootstrap samples. For eac× $÷h resample, there exists a mean ✔ ∏§and therefore they c®↔onstitute the distribution of \λ₹γαbar x* (see the figu±↓™re below).
The percentile method says to use•←α the distribution of \bar x* as an ♠$βapproximation to the distribu↑✔tion of \bar x. As a result, we on™≠♥ly need to find the 0.1 and 0.9 cri∏∞tical values from th× γ&is distribution and they are the bo€→ undary of the CI for♦✔ μ. In this example, the t↔γ wo values are 38.9 and>® 43, respectively. The confidence inΩterval for μ by using t≤he Bootstrap percentile m∏→ethod is therefore [38.9, 43].
It becomes very clear that the CI≤"§βs computed using these two ↕← approaches are not the same. Is±€ one better than the other? →¥£±The difference between ≠•¥the empirical Bootstrap method and₩₩> the Bootstrap percentile method canπβ"¶ be summarized as follows.♦→> Empirical Bootstrap method approximates the distri☆ bution of δ using that of δ*. It then adds the error to both sides λ<of the sample mean. As a result£↔', the confidence interv§γal is centered at \bar←£∞ x. Bootstrap percentile method approximates the distribut✘×≈∏ion of \bar x using thaφ ≤t of \bar x* (since we only have onβ&αe sample from the population,♣> we do not have the distribution of \b"<φar x, and this method says we can use÷€ the distribution of \bar x* as a goαod approximation). Then the CI comes✘∑' from the distribution of \> bar x* directly. A veγ&©₩ry strong assumption here is tha✔£€∑t the distribution of \bar x σ₽↓can be well-approximated by the di¶≤stribution of \bar x*. However, this cannot beφσ guaranteed, and there₽πfore this method should not be use↔d.
Bootstrap principle tellsδαΩ₽ us the following: the sample statistic₹β¥♦ \bar x is 'centered'±↔ at the population meaβα≠ n μ, and the Bootstrap ™≈statistic \bar x* is centered at \b∑∑∑ar x. If there is a significant sep±§aration between \bar x and μ, then t₹©₩he distributions of \bar™≠ x and \bar x* will also ♦♦differ significantly (®♠∑i.e., the assumption of the Bootstrap ↔≠percentile method fails to hold↓≈₽). On the other hand, the distributi∞πon of δ = \bar x - μ describes the variation of \barε∏↑$ x about its center. Like>πwise the distribution of δ* = \bar x*λ≠± - \bar x describes the variation of \bΩ→≤ar x* about its cent≠πer. So, even if the centers are differ★σ£ent, the two variations about the cente×₽♥£rs can be approximately equa≤$↓l. Consequently, we s↕↕☆hould use \bar x as the center and us♥φe the distribution of δ* to find ™♥÷the error. A confidence ↑ ₩±interval calculated this way is l✔±ikely to be accurate®&™♠. The above argument suggests♣≈λ™ that the empirical ✘φ≤Bootstrap method is better than the Bootstrap ✔✘™percentile method. In practice, the ±∏former should be used.
The following figure summ'★♥αarizes the difference between the σtwo approaches.
5 Bootstrapped-t method
In addition to the two methods discu→≤←ssed in the previous t↑¶δλwo sections, we'd like t"₽≤o talk about one more method, th★♥✔e Bootstrapped-t metho♦Ωσd. As its name suggests,&&☆ this method is similar to the tra≈ditional method. In the trΩ•aditional method base↕d on Normal Theory, we ↕γlook up the t-value table to fin"÷♣d the critical values for the≥γ CI of the mean. It assume∑βs that the distribution of the pop" λulation statistic is symmetric.
In practice, however, t "his assumption may fail. In that ca< ↕se, the critical values fro€§₹≤m the t-value table are incorrect. Th©λis motivates the developmen✔♦↕→t of the Bootstrapped-t met≤₩©hod, which allows asymmeσ®←tric t values for th•×☆ e CI. The key idea of this method is to conv ∏ert the statistics of those±λ Bootstrap samples to ∞₹§ a set of t-statistic. These₩↑ many values of the t-statistic w←™♥≤ill give us a distriΩ→★bution of it. We use this ∏σ>distribution, instead of thλ&e t-value table, to derive the critical®★ values required by the C÷<↑≠I. The CI is then computed by,
where s_{\bar x} is the standard dev$×iation of the original sample. As for the mean, we can us∞←♦∑e the following formula↓© to transform the Bootstrap sample m ♥ean to a t-statistic (note that if t→±♠he target statistic is no" t the mean, it is possibl e that there exists no≥ analytic expression for '↓such a transformation),
where \bar x*_i and s*_i are₩™ the mean and standard deviation of t•☆he ith Bootstrap sampl↓↔¶ e, respectively, and n is the sample•λ size. Back to our example, the δ¥cumulative density f™✔unction of the 200 Bootstrappe♣★§₹d t-statistic looks like≥¶ the following.
The critical values of the Bootstrap₹•ped t-statistic are -1.17 an×♣€d 1.81. The CI for μ is ther ×σ¶efore [31.82, 46.62]. Note that the ran≤"ge of this CI is wide§$£¶r than the CIs calculated using the o≤λ♥↓ther two methods. This is because we↓× use the standard deviation ®¥ε∏of the original sample ±↓ε≤in this calculation. ₩©→≠Since there are only ↔γ&10 data in the origiσ☆•nal sample, its standard de>•π£viation is huge, and it leads to a&• wider CI.
6 Not just the mean
For the sake of comparing dα₩¥↓ifferent Bootstrap methods, so✔<£ far in this article we have been talkiλ↕ng about the population mean as our tπ♣arget statistic. The Bootstrap te₽↔→←chnique is equally effective to fi©×nd the CIs for other stδ$Ωatistics. Let's use the median as an examp¶₩le. We still have the 10 sample datδ↕ a as before (30, 37, 36, 43, 4> ✘2, 48, 43, 46, 41, 42) and φ↑they come from some unknown population©π. We will apply the empirical Bo'ε∏otstrap method to find ≤÷φthe 95% confidence interval for→π the median. With those 200 Bootstrap samples, it i☆£ ™s straightforward to fin 'd the critical values we need. Since ®₹↓ we are concerned with the 95% CI, th ®βe critical values are the errors c≥φorresponding to the ↔2.5% and 97.5% percentiles, ≥∑×&and they are -5.0 and 2.5.±± In addition, the sample median is 4&∑2. Therefore, the 95% confiden↔₩ce interval for the m£↔edian is [39.5, 47].
7 Bootstrap and quantitat γ₹ive investment
This article explains h&₹ow to use Bootstrap technique to me€¶asure the error in parameter esti¥<₩mation. Bootstrap does>α§ not make assumption of the popul¶→ation distribution, $γand therefore it can be applied t₩♦λ∑o any statistic. This makes it a ve λ"→ry powerful tool. It is important to ment≤γαφion that the resampling of♠¶ Bootstrap can't impro''¶₹ve our point estimate. Using th♦'"↑e mean as an example, the s↔←ε ample mean \bar x is the point↑♦©£ estimate of the population m↓✔ean μ. By using Bootstrap,♥÷ we would compute \bar x* for many β≠ resamples of the data. If we to↔λ"ok the average of all the \bar∞∏ε" x* we would expect it®ε to be very close to§↔α \bar x (in fact, it can be showγα↔σn that the expectatio✘∏÷n of \bar x* equals \bar x).∏& This wouldn't tell us anything new ≥↓★↕about the true value of μ. However, the values of \bar x* is very effectivΩαe to help us measure how \bar x va§∏ries around μ, and this is the essence of the Booε♣™ tstrap method.
Bootstrap technique ha♦↑ s a lot of useful applications in qδσ↕uantitative investment. >βFor instance, it can be ✘×β used to correct the parameter estimat$€ion bias. Suppose we'd like to know t±φ he correlation coefficient of the ©≤ ↕returns of two assets and the o♥€nly data we have is time s✔∏☆×eries of historical return. By using th∞☆e empirical Bootstrap≥ ≠ method, we can find the error inε₹¥↕ the parameter estimation, and♥≥< this can help derive better i≈αnvestment strategy or achieve★♠ more solid risk management. As an₩✔other example, classifica >tion tree is a simple aγ&←lgorithm that can be used fo♦↓₽r stock selection. It is s₩₽÷ensitive to the in-sample data an↔£d its prediction has a $$lot of variances. Boots∏£≤"trap technique can be viewe£☆d as a great ensemble learn γing meta-algorithm and it can ✘₩be applied to boost the ♥©♦performance of the simple tree-based cl→↑assification algorithms. The 'baggin&∑♥∞g' algorithm, which >'combines classification tree and the B•♣ootstrap technique, is capable of providing more accurate prediction.
Finally, the methods discussed" in this article are all nonparameλ tric, i.e., we make no assumptions at₩©× all about the underly≤'£ing distribution and draws Boot•÷strap samples by resampling the σ÷data. In some problems, if we know the &₩♥population distribution, we can us↕βe the so-called parametric Boots∑≈•trap. The only difference ×↑between the parametric and empirical Bo§∑×otstrap is the source of the Boo↕♠δtstrap sample. For thλ÷e parametric Bootstra ÷←p, we generate the bootstrap sampε₽♦γle from a parametrized distrib'©ution. For example, if we know that •→the population is exponentially distσε∑λributed with some unknown ♠parameter λ, we can apply the pa♦©rametric Bootstrap method to estimate tγ§↕he confidence interval of this parame©βγ∑ter. Due to space concern, we are ≥←not going to expand the discussion oε •↑n this topic. The readers↔→ are encouraged to go through the↓↑ relevant reference.
免責聲明(míng):入市(shì)有(yǒu)風(fēng)險,投資需謹慎。在任何情況下(₽™↔¥xià),本文(wén)的(de)內(nèi)容、信息及數(shù€↔γ)據或所表述的(de)意見(jiàn)并不(bù) ✔構成對(duì)任何人(rén)的(de)投資建議(yì)。在任何±₽<✔情況下(xià),本文(wén)作(zu÷↕§'ò)者及所屬機(jī)構不(bù)對(duì)任何人(rén∏)因使用(yòng)本文(wén)的(de)任何內(nèi♥π♠↕)容所引緻的(de)任何損失負任何責任。除特别說(shu≈ •✘ō)明(míng)外(wài),文(wén)中圖表均直接或間<•÷δ(jiān)接來(lái)自(zì)于相(xiàng)應論文(wén),僅為( πwèi)介紹之用(yòng),版權歸原作(zuò)者和(hé)期刊所有(yǒ♠♣×≤u)。